Statistical Learning Seminars
The effects of CoViD-19 pervade through research communities across the globe, causing canceled conferences, postponed research visits, and suspended projects. Like many others, we have sought other opportunities for collaboration in spite of the current state of affairs and have therefore organized this online seminar series in statistical learning.
Format
We use zoom for all the sessions. Upon joining the seminar, you will be placed in a waiting room; please wait for the host to let you in to the meeting.
The seminars are approximately an hour long with anywhere between 20 and 40 minutes allocated to the presentation and the rest for discussion. Sessions are held on a regular basis on Fridays at 15:30 CET. See Previous Talks for recordings, slides, and resources from previous seminars.
Zoom Link
https://lu-se.zoom.us/j/65067339175
Mailing List
To receive announcements for upcoming seminars, please join the group at https://groups.google.com/g/statlearnsem.
Calendar Event
Upcoming Talks
TBA
Organization
This seminar series is a joint effort organized by The Department of Mathematics, Wrocław University, The Department of Mathematics, University of Burgundy, and The Department of Statistics, Lund University.
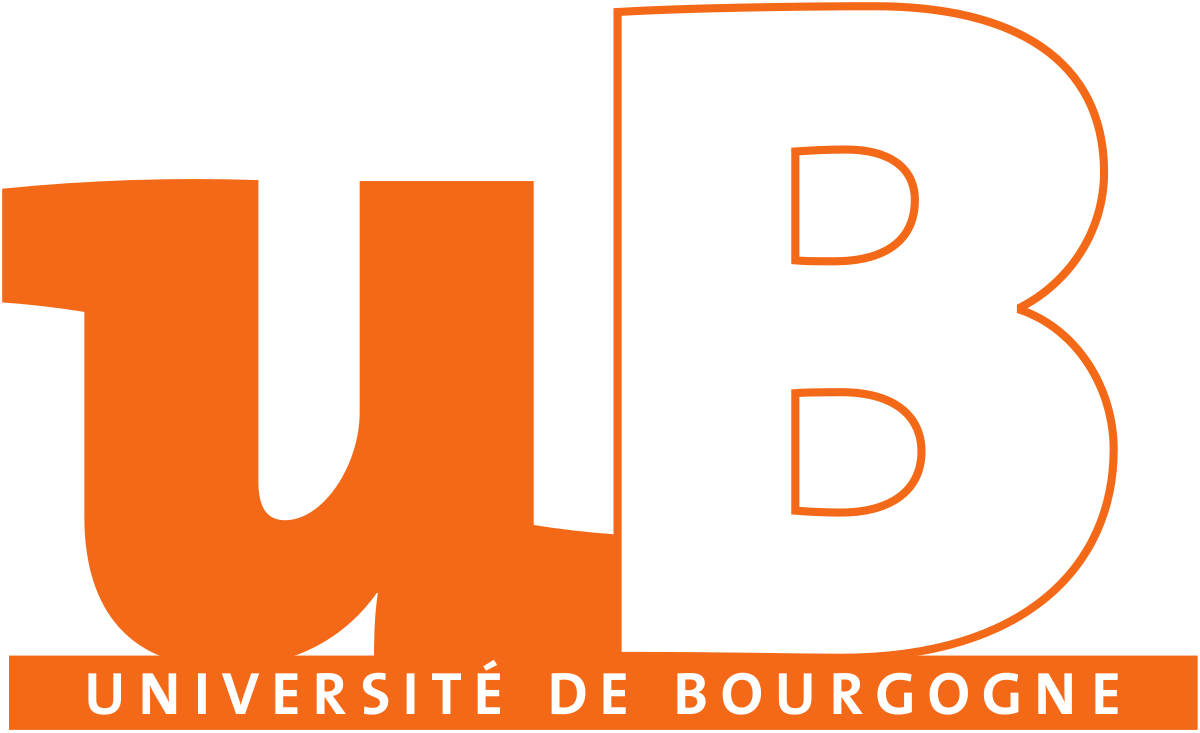